Reducing Inventory and Improving Service: Unveiling Overlooked Data Driven Strategies
- Daniel Vanden Brink
- Feb 22, 2024
- 5 min read
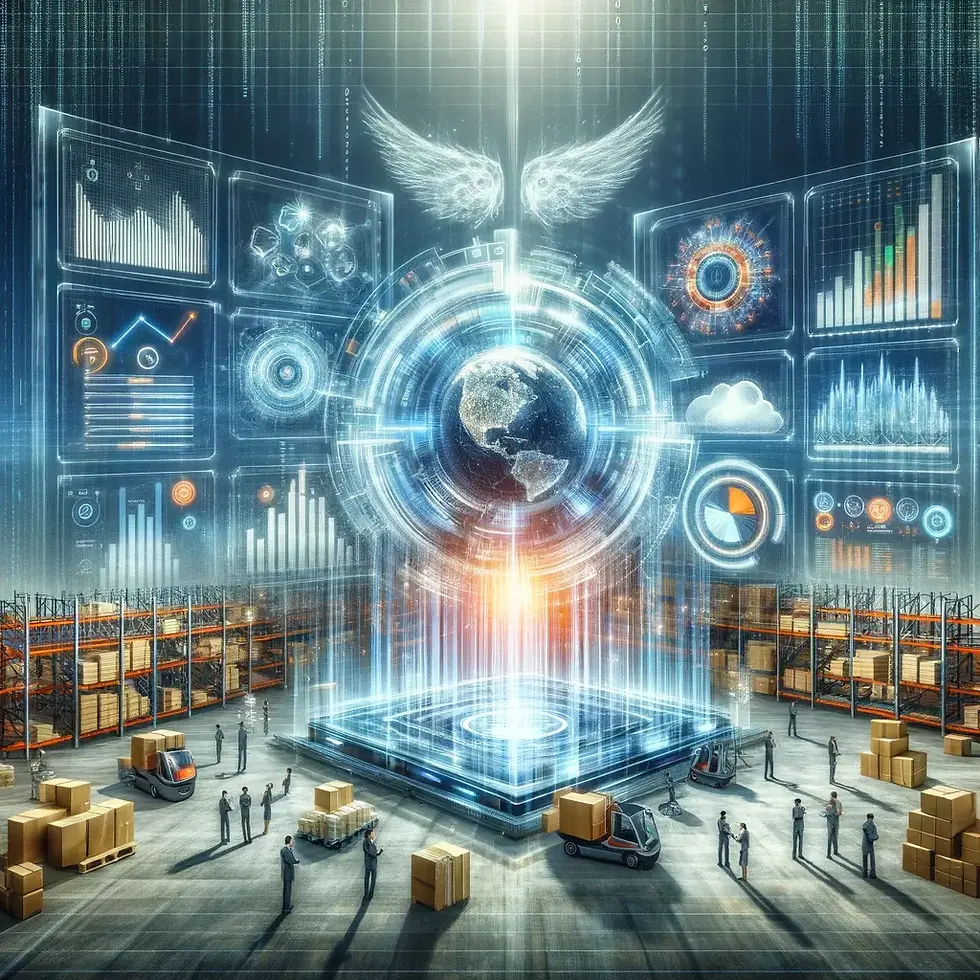
Reducing Inventory and Improving Service: Unveiling Overlooked Data Driven Strategies
In the wake of the COVID-19 pandemic and subsequent disruptions, companies worldwide have grappled with inventory management challenges. While recent events have highlighted inventory issues, inventory management has always been and continues to be challenging for many companies. Forecast accuracy has always been the primary focus for companies trying to improve inventory and service levels, but other levers within supply chain planning are generally ignored or overlooked. This article will review 3 main areas often overlooked that companies should evaluate as part of the inventory improvement programs.
Forecasting Enhancements
Traditionally, forecasting has been the cornerstone of inventory management strategies. The enhancements provided by AI/ML are substantial and will not be the focus of this article, rather the focus will be on nuances that are often disregarded. One such aspect is risk pooling, a pivotal factor in inventory management. Risk Pooling takes advantage of how standard deviations are added together. For instance, consider two customers, A and B, with respective demands of 100 and 50 units, each accompanied by their standard deviations of 25 and 20. The coefficient of variation (CV), which measures demand variability relative to the actual demand is 0.25 for customer A and 0.4 for customer B. When these demands are aggregated together to 150, the resulting standard deviation, assuming independence, is calculated by summing the squares of individual standard deviations and taking their square root:
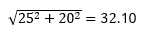
therefore the combined CV is now lowered to 32.10/150 or 0.21.
However, real-world demand patterns often exhibit correlations between customers or across time periods, contrary to the assumption of independence which is assumed in almost all inventory calculations. By incorporating a covariance coefficient (COV), which denotes the relationship between demands, the calculation of combined standard deviation becomes more nuanced. A negative COV implies an inverse relationship between demands, leading to a reduced combined CV and enhanced risk pooling benefits. For example, if we did the same calculation but now let’s put in a COV which measures the relationship between customer A and B’s demand of -125 which implies that if customer A’s demand goes up customer B’s demand goes down. Now the calculation for the standard deviation of the combined demand is:
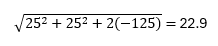
and a new CV is 0.15 – lower than if we assume independence and would result in less safety stock inventory vs. the standard approach to pooling demand. Conversely, a positive COV would increase the CV, diminishing the effectiveness of risk pooling and resulting in poorer service if the traditional approach was being utilized. This relationship is also important when calculating demand lead time standard deviation utilized when calculating safety stock.
The generalized matrix form of the equation above is:

These matrices can become very large, but memory and computing power are no longer a limit and are easy to do. Estimating and understanding the COV matrix between all customers and time periods as part of your demand forecasting process can have an impact on a company’s inventory levels.
The other area of improvement is also around estimating forecast error or standard deviation. Most companies assume that the standard deviation is homoscedastic or simply that the standard deviation is constant across the forecast, which again is generally not true. Understanding how your forecast error changes with time can lead to better inventory policies.
Inventory Modeling
While contemporary supply chain software offers rudimentary safety stock and EOQ calculations, their simplistic nature often overlooks intricacies. Distinguishing between cycle service level and fill rate elucidates distinct facets of inventory performance. Below are the differences between both approaches:
Cycle Service Level
o Cycle service level refers to the percentage of customer orders that are fulfilled within a specified time frame, typically within a single order cycle.
o It measures the ability of a company to meet customer demand promptly and reliably.
o It considers whether customer orders are fulfilled on time, regardless of the quantity ordered.o Formula: (Number of orders delivered on time / Total number of orders) * 100
Fill Rate
o Fill rate measures the percentage of customer demand that is met immediately from stock, without backorders or stockouts.
o It specifically focuses on the quantity of items delivered to customers from available inventory.
o Fill rate takes into account whether the ordered quantity is fully available at the time of order fulfillment.
o Formula: (Quantity of items delivered / Quantity ordered) * 100
Aligning safety stock calculations with the correct service level policy is paramount, as discrepancies can lead to either excess inventory or compromised service quality. Cycle stock is typically what most textbooks and customers are utilizing, but fill rate is often the policy that companies are measuring and want to adhere to.
Additionally, the assumption of normal demand distribution warrants scrutiny, particularly in scenarios of high coefficient of variation (CV especially when the CV > 43% and over 1% of your implied demand is negative which is false. Alternative distributions such as Poisson or truncated normal offer more robust representations when CVs are higher, essential for accurate safety stock estimations.
Finally, end-to-end inventory optimization is an excellent way to understand how demand uncertainty impacts inventory and how one can optimally position inventory to manage that uncertainty while minimizing inventory costs. In the figure below, we can see that the main drivers believed to be impacting inventory were not the key drivers. Rather, the positioning and synchronizing across the entire supply chain, and inventory could be dramatically reduced.
Multi-echelon Inventory Optimization is an excellent application to leverage in understanding inventory drivers within your supply chain.
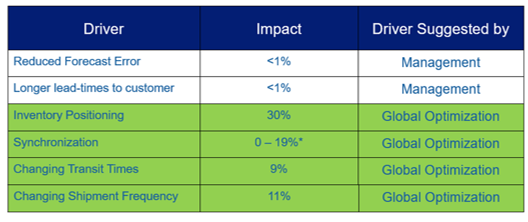
Lead Time Prediction with AI/ML
Accurate lead time estimation lies at the crux of effective inventory management and material planning. Yet, conventional approaches relying on vendor-provided estimates often falter. Leveraging AI/ML models facilitates dynamic lead time predictions, accounting for fluctuations induced by holidays, capacity constraints, or supply chain disruptions. Notably, these models ensure that planning systems properly synchronize material arrivals, lowering inventory holding costs and streamlining production processes. Moreover, in scenarios of novel product introductions or complex bills of materials (BOMs), ML algorithms excel in extrapolating lead times based on component characteristics, even in the absence of historical data. One customer that implemented this type of solution saw a 5% reduction in inventory in the 1st quarter that was deployed and projected to lower inventory with an additional 20% reduction projected.
Leveraging ML for Inventory Monitoring
ML emerges as a potent tool for preemptive inventory management, enabling early detection of anomalies and forecasting inventory trends. By discerning underlying patterns within transactional data, ML algorithms facilitate proactive decision-making, thereby averting stockouts or inventory surpluses. Moreover, the insights gleaned from these models furnish invaluable inputs for root cause analysis, fostering a culture of continuous improvement within supply chain operations.
Conclusion
While forecast accuracy remains pivotal, unlocking the full potential of supply chain optimization demands a multifaceted approach. By delving into overlooked areas such as risk pooling, precise inventory modeling, and dynamic lead time prediction, companies can realize substantial gains in both inventory efficiency and service excellence. Embracing AI and ML technologies not only augments forecasting capabilities but also heralds a paradigm shift towards proactive and data-driven supply chain management.