Inventory Rebalancing Optimization
- Rami Nasrallah
- May 13, 2024
- 2 min read
CPG company automates and optimizes transshipment decision-making across its main warehouses to increase consumer service levels while reducing logistics costs.
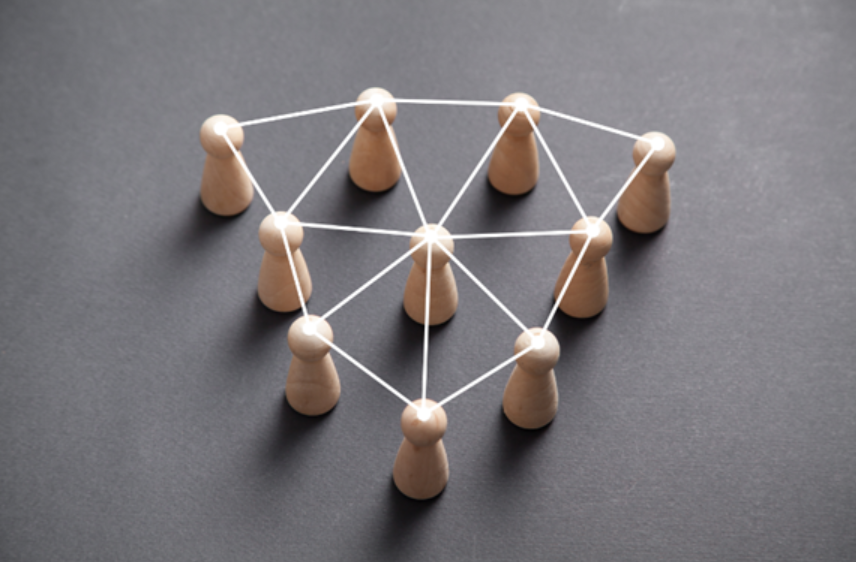
Background:
A leading global CPG company with an annual revenue exceeding $30 billion fulfills demand in the US through its strategically located network of multiple main distribution centers (DCs). The company offers a portfolio of over 2,000 diverse products, including regular stock, seasonal items, and unique marketing display products. Currently, the supply chain team manually calculates and executes transshipments for these products across the network as part of an Inventory Rebalancing process. This process, which requires a minimum of 140 human-hours per week, serves as the final line of defense to maintain service levels. Recognizing the need for a more efficient approach due to the required response time, the complexity of decision variables, and fluctuating demand, the company sees an opportunity to revolutionize the process.
Challenges:
In defining the requirements for the automated AI tool, several significant challenges emerged:
Multiple Data Sources - The company utilized both SAP and Kinaxis systems, necessitating the tool to seamlessly incorporate data from these sources and understand the decisions made by existing planning systems.
Non-Standard Process – The diverse range of product types (Turn, Seasonal, Campaign, etc.) required different considerations due to their unique behaviors. Additionally, each planner had a distinct approach to calculating and executing transshipments.
Many Variables/Trade-Offs – Various factors required consideration, including lead times, logistics costs, demand types, and types of excess and stocks. However, current decisions were often made for simplicity's sake rather than optimizing across all available factors.
Impact Metrics – The company lacked a tracking system or historical analysis of the inventory rebalancing process’s impact on service levels, hindering the ability to drive improvements.
Solution:
An optimization model was developed to revolutionize the process, the company’s first supply chain decision intelligence tool. This model seamlessly integrates data from various sources, analyzes the entire range of products daily, and generates optimized stock transfer orders (STOs), seamlessly incorporating them into the company's SAP system.
The tool's design and logic not only captured and refined but also evolved expertise from the company’s supply chain team. Establishing a standard approach, the model covered all product types with distinct behaviors through specific parameters or constraints. Early and high adoption was achieved through quick and frequent feedback loops between planners and the development team before the initial release.
Impact analysis and dashboards were developed and updated weekly to monitor successful adoption, impact of decisions on the network and logistics costs. The analysis has already been utilized to provide insights for upstream and downstream business processes as well as for continuous improvement of the model and future releases.
Benefits:
Implementation resulted in some astounding improvement, and the company is planning expansion across markets. In the first year, the automated process successfully transshipped $1.2 billion of GSV, yielding several benefits:
$18 million in incremental earnings.
A 30% reduction in logistics costs, amounting to $3.5 million in savings, and a reduction of 13.4 kilotons in carbon emissions.
A 75% reduction in decision-making time.
Conclusion:
The company’s first decision intelligence tool has paved the way for more data-driven models and become a flagship for the potential of advanced analytics to help daily ways of working. The Inventory Rebalancing process has been revolutionized, enabling the company to enhance its end-to-end process efficiency, as well as providing valuable insights for other processes. The tool is set up for scalability and continuous improvement for future growth and enhancements.